Leveraging Behavior Analytics for AML Compliance: A Technical Insight
In the battle against financial crimes, Anti-Money Laundering (AML) compliance is a critical line of defense. Financial institutions are mandated to implement robust AML programs to detect, prevent, and report suspicious activities. Traditional rule-based systems, while effective to an extent, often fall short in identifying sophisticated money laundering schemes. This is where behavior analytics offers a more dynamic and intelligent approach to AML compliance. In this blog post, we delve into the intricacies of behavior analytics, its significance in AML compliance, and how it is utilized to enhance the detection and prevention of financial crimes.
What is Behavior Analytics?
Behavior analytics involves collecting and analyzing data on the behavior of individuals and entities to identify patterns, anomalies, and trends. In the context of AML, behavior analytics examines the actions and transactions of customers to uncover suspicious activities that may indicate money laundering or other financial crimes. By leveraging advanced technologies such as machine learning, artificial intelligence, and big data analytics, behavior analytics provides a deeper and more nuanced understanding of customer behaviors compared to traditional methods.
Why is it Important in AML Compliance?
The importance of behavior analytics in AML compliance cannot be overstated. Here are key reasons why it is crucial:
- Enhanced Detection of Anomalies: Behavior analytics can identify subtle and complex patterns that may be indicative of money laundering activities, which traditional rule-based systems might miss.
- Adaptive Learning: Unlike static rule-based systems, behavior analytics solutions can learn and adapt over time, improving their accuracy and effectiveness in detecting suspicious activities.
- Reduced False Positives: By providing a more nuanced analysis of behaviors, behavior analytics can significantly reduce the number of false positives, allowing compliance teams to focus on genuine threats.
- Comprehensive Risk Assessment: Behavior analytics offers a holistic view of customer activities, enabling a more comprehensive risk assessment and proactive measures to prevent financial crimes.
How Does Customer Behavior Analysis Work?
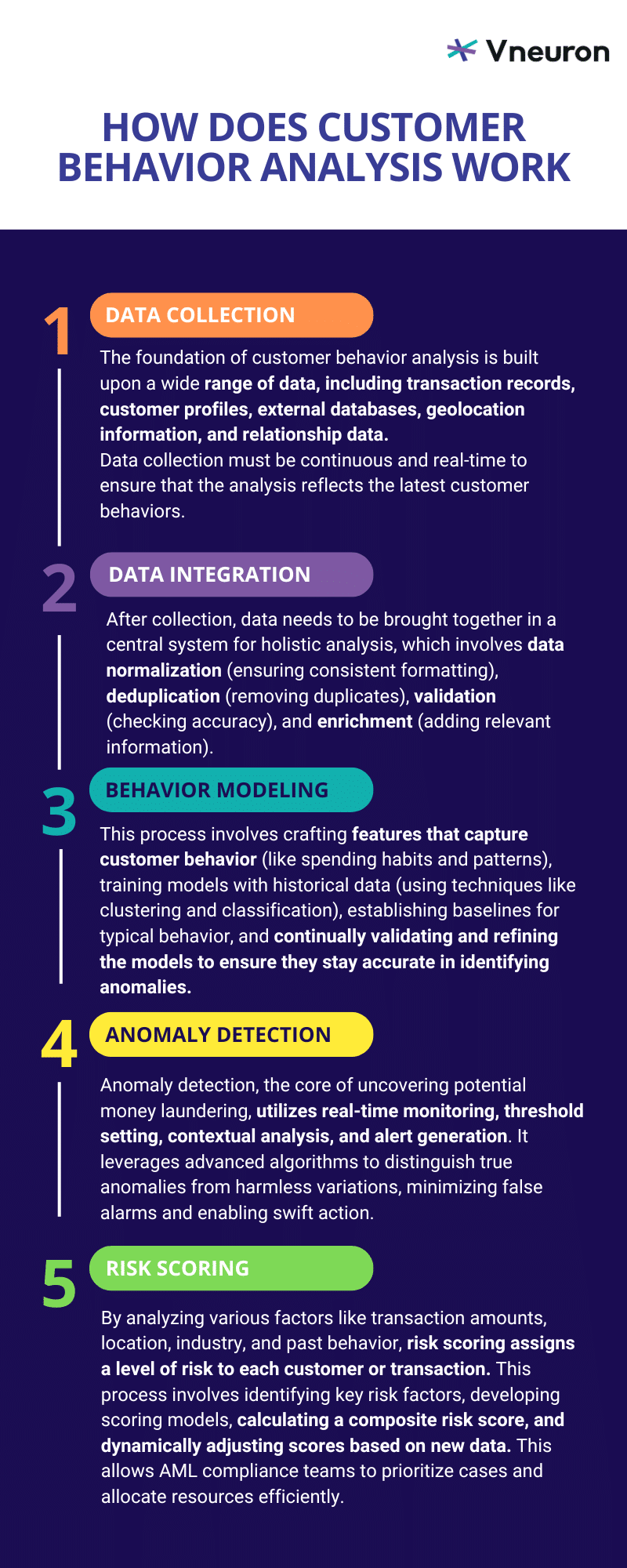
Customer behavior analysis in AML compliance is a multi-faceted process that involves several detailed steps. Each step is crucial for building a comprehensive and effective system to detect and prevent money laundering activities:
1. Data Collection
The foundation of customer behavior analysis lies in extensive data collection from a multitude of sources. These sources include, but are not limited to:
- Transaction Records: Data on all transactions made by customers, including transaction amounts, frequencies, types (e.g., deposits, withdrawals, wire transfers), and timestamps.
- Customer Profiles: Detailed information about customers such as personal identification details, account types, historical data, and demographic information.
- External Databases: Information from external sources such as credit bureaus, sanction lists, politically exposed persons (PEP) lists, and other relevant databases that provide additional context.
- Geolocation Data: Information about the geographic locations where transactions are conducted. This can include IP addresses for online transactions and physical locations for in-person transactions.
- Relationship Data: Data illustrating relationships and connections between different entities, which can be critical for identifying networks of suspicious activities.
Data collection must be continuous and in real-time to ensure that the analysis reflects the latest customer behaviors.
2. Data Integration and Cleaning
Once collected, data must be integrated into a centralized system where it can be accessed and analyzed holistically. This involves:
- Data Normalization: This step is about ensuring that data from different sources conform to a standard format. This includes standardizing dates, currencies, and other relevant fields.
- Data Deduplication: Identifying and removing duplicate records is crucial in data cleansing to avoid redundant analysis.
- Data Validation: This step of the process involves checking for and rectifying inaccuracies or inconsistencies in the data. This might require cross-referencing with external databases or using automated validation rules.
- Data Enrichment: Including additional information relevant to the analysis, such as external risk scores and demographic information can improve the results of the whole process.
Data Integration and Cleaning ensures the data’s accuracy, consistency, and readiness for analysis, which is crucial for the reliability of subsequent behavior modeling and anomaly detection.
3. Behavior Modeling
In behavior modeling, advanced algorithms and machine-learning techniques are used to create detailed profiles of customer behavior. This involves:
- Feature Engineering: This step involves identifying and creating features that best capture the essence of customer behaviors. Features can include transaction frequency, average transaction value, transaction types, and changes in transaction patterns over time.
- Model Training: At this stage, historical data is leveraged to train machine learning models. Common techniques include clustering (e.g., K-means clustering) to group similar behaviors and classification (e.g., logistic regression, decision trees) to predict the likelihood of suspicious activity.
- Pattern Recognition: This involves establishing baseline behavior patterns for each customer or customer segment. These patterns serve as reference points against which new behaviors are compared.
- Model Validation: Continuously validating and refining models using new data is important to ensure their accuracy and effectiveness in identifying genuine patterns versus anomalies.
Behavior models are dynamic and evolve as more data is collected and analyzed, allowing them to adapt to new patterns of legitimate and suspicious activities.
4. Anomaly Detection
Anomaly detection is the crux of identifying potential money laundering activities. It involves:
- Real-Time Monitoring: This relies on continuously monitoring transactions and behaviors in real-time to detect deviations from established behavior models.
- Threshold Setting: This step includes defining thresholds for various metrics (e.g., transaction amounts, frequency of transactions) that, when exceeded, indicate potential anomalies.
- Contextual Analysis: Evaluating anomalies within the broader context. For instance, a large transaction might be normal for a high-net-worth individual but suspicious for an average customer.
- Alert Generation: This step is one of the most critical steps in anomaly detection, which includes triggering alerts for further investigation when anomalies are detected. These alerts are prioritized based on the severity and risk associated with the detected behavior.
Effective anomaly detection relies on sophisticated algorithms that can differentiate between genuine anomalies and benign deviations, reducing false positives and ensuring timely intervention.
5. Risk Scoring
Risk scoring assigns a risk level to each customer or transaction based on the analysis performed. This involves:
- Risk Factors Identification: This step is about identifying key risk factors such as transaction amounts, geolocation of transactions, involvement in high-risk industries, and historical behavior patterns.
- Scoring Models: This involves developing and applying scoring models that quantify the risk associated with each factor. These models often use techniques such as logistic regression, decision trees, or ensemble methods.
- Composite Risk Score: Calculating a composite risk score that aggregates the individual risk factors into a single, actionable metric is crucial at this stage. Higher scores indicate a higher likelihood of money laundering.
- Dynamic Adjustment: Continuously adjusting risk scores as new data is collected and analyzed is essential to ensure that risk assessment remains current and accurate.
How AML Compliance Solutions Use Behavior Analytics in Compliance Modules
AML compliance solutions incorporate behavior analytics into their compliance modules to provide a robust framework for detecting and preventing financial crimes. Here’s how:
1. Transaction Monitoring:
- Enhanced Contextual Analysis: Behavior analytics in transaction monitoring systems go beyond simple rule-based detection. They analyze transactional context, including transaction patterns, frequencies, amounts, and relationships between entities. Advanced algorithms can detect anomalies indicative of money laundering schemes, such as structuring or rapid fund movements across borders.
- Machine Learning Models: Transaction monitoring systems utilize machine learning models to adaptively learn and identify evolving patterns of suspicious activity. These models can detect anomalies that may not be apparent through traditional rule-based approaches, improving detection accuracy.
2. Customer Risk Profiling:
- Dynamic Risk Assessment: Behavior analytics continuously assess customer behavior to dynamically update risk profiles. This involves analyzing various factors such as transaction history, frequency, size, and geographic locations. High-risk behaviors trigger automated alerts, prompting enhanced due diligence processes for those customers.
- Predictive Analytics: Advanced analytics techniques, including predictive modeling, enable AML solutions to anticipate potential risks based on historical behaviors. By identifying patterns indicative of illicit activities, predictive analytics assist in proactive risk management and compliance efforts.
3. Alert Generation and Management:
- Risk-Based Prioritization: Behavior analytics assigns risk scores to detected anomalies, facilitating the prioritization of alerts based on their severity and likelihood of being associated with financial crimes. This ensures that compliance officers focus their attention on the most critical cases, improving operational efficiency and reducing response times.
- Automated Workflow: AML solutions automate the workflow for alert generation, investigation, and resolution. Integration with case management systems streamlines the process, allowing for efficient collaboration among compliance teams and ensuring timely resolution of flagged activities.
4. Regulatory Reporting:
- Comprehensive Reporting: Behavior analytics generates comprehensive reports for regulatory authorities, providing detailed analysis and evidence of suspicious activities. These reports include transaction histories, anomaly detection results, risk assessments, and actions taken by the institution to mitigate risks.
- Regulatory Compliance Automation: AML solutions automate the generation and submission of regulatory reports, ensuring timely compliance with regulatory requirements. Behavior analytics plays a crucial role in ensuring the accuracy and completeness of these reports by providing granular insights into detected anomalies.
5. Continuous Improvement:
- Feedback Loop: Behavior analytics systems incorporate a feedback loop mechanism to continuously improve detection capabilities. They learn from past investigations, including false positives and false negatives, to refine detection algorithms. This iterative process enhances the accuracy and effectiveness of the system over time.
- Model Retraining: Machine learning models used in behavior analytics undergo periodic retraining with updated data to adapt to evolving threats and regulatory changes. This ensures that the system remains resilient against emerging money laundering techniques and maintains compliance with evolving regulatory standards.
Behavior analytics represents a significant advancement in AML compliance, offering a sophisticated and adaptive approach to detecting and preventing financial crimes. By analyzing customer behaviors and identifying anomalies, behavior analytics enhances the effectiveness of AML programs, reduces false positives, and provides a comprehensive view of risks.
As financial crimes become increasingly sophisticated, the integration of behavior analytics into AML compliance solutions is essential for staying ahead of illicit activities and ensuring the integrity of the financial system.
Experience the power of behavior analytics in AML compliance with Vneuron! Our AML compliance platform leverages cutting-edge behavior analytics technology to safeguard your institution against financial crimes.
Schedule a demo today to see how Vneuron can strengthen your compliance efforts and protect your organization’s reputation.